Page • The Dakota project delivers both state-of-the-art research and robust, usable software for optimization and UQ. Broadly, the Dakota software's advanced parametric analyses enable design exploration, model calibration, risk analysis, and quantification of margins and uncertainty with computational models. The Dakota toolkit provides a flexible, extensible interface between such simulation codes...
Search
CASL Manual
Page • Adams, B.M., Coleman, Kayla, Hooper, Russell W., Khuwaileh, Bassam A., Lewis, Allison, Smith, Ralph C., Swiler, Laura P., Turinsky, Paul J., Williams, Brian J., "User Guidelines and Best Practices for CASL VUQ Analysis Using Dakota, Sandia Technical Report 2016-11614 (also CASL-U-2016-1233-000), September 2016.
Citing Dakota
Page • Please cite the Dakota User's Manual (or other appropriate manual) for the version you used (see SAND Reports). For example: B. M. Adams, W. J. Bohnhoff, K. R. Dalbey, M. S. Ebeida, J. P. Eddy, M. S. Eldred, R. W. Hooper, P. D. Hough, K. T. Hu, J. D. Jakeman, M....
Contributing to Dakota
Page • Our open source software benefits greatly from the contributions of its user community. Ways to contribute include: Participate in Dakota's GitHub Discussions by asking and answering questions, reporting bugs, suggesting new features, or sharing ways you've used Dakota in your work.Properly cite Dakota when you use it in your work.Port...
Contributors
Page • Potential contributors to Dakota should review the information on Feature Additions regarding means and terms of contribution. Historical Dakota Authors Individuals who have contributed directly to Dakota's core since its inception in 1993, including source, examples, and infrastructure. Other current developers are listed at About - Team. Format: author (organization...
Dakota 6.16
Post, May 17, 2022 • Highlight: Multifidelity UQ Methods Dakota 6.16 significantly extends capabilities for multifidelity uncertainty quantification (MF UQ) based on random sampling, including iterated versions of approximate control variate (ACV) and multifidelity Monte Carlo (MFMC), new solution modes (online pilot, offline pilot, and pilot projection), new final statistics goals supporting estimator selection and tuning, online cost recovery...
Dakota 6.17
Post, November 15, 2022 • Highlight: Integrated User Manual Dakota 6.17 includes a beta version of a new Sphinx-based integrated user manual. It aggregates content from the historical User’s, Theory, and Reference manuals, as well as the Dakota website. Feedback on this new compendium is invited. Enabling / Accessing: Primarily from https://dakota.sandia.gov, also in <dakota_src>/docs/user....
Dakota 6.18
Post, May 24, 2023 • Highlight: Generalized Approximate Control Variate Method for Multifidelity Sampling Dakota can now search over directed acyclic graphs to identify the best model inter-relationships for multifidelity sampling. Enabling / Accessing: As part of the approximate_control_variate (ACV) method for multifidelity sampling, the new search_model_graphs option activates the generalized ACV capability that identifies...
Dakota 6.19
Post, November 14, 2023 • Announcing Dakota Version 6.19 Dakota 6.19 is officially available for download. Highlight: New sampling-based method for Sobol’ main effects Based on [LM16], Dakota can now obtain estimates of first order Sobol’ indices (main effects) from sampling studies. Previous versions of Dakota could estimate main and total effects using a “pick...
Dakota 6.20
Post, May 15, 2024 • Announcing Dakota Version 6.20 Dakota 6.20 is officially available for download. Highlight: Multilevel Best Linear Unbiased Estimator (ML BLUE) ML BLUE ([SU20]) is a new multifidelity sampling-based approach for UQ, distinguished from other estimators through its use of sample allocations based on model groupings. It has motivated a number of...
Dakota 6.21
Post, November 14, 2024 • Announcing Dakota Version 6.21 Dakota 6.21 is officially available for download. Highlight: Numerical Robustness for ML Blue The multilevel best linear unbiased estimator (ML BLUE) now uses truncated SVD for all matrix solutions (previously handled by Cholesky factorization with equilibration), enabling more robust solution recovery for resouce allocations and final...
Dakota JAGUAR (Legacy GUI)
Page • JAva GUi for Applied Research (JAGUAR) was developed as a graphical user interface (GUI) for earlier Dakota versions (circa 5.x, 6.x). This download page is provided as a historical reference only. Current Dakota users should instead use the GUI available for download alongside (or packaged with) the Dakota version in...
Downloads
Page • Downloads of Dakota are available on our GitHub Releases page. Supported Platforms Downloads are provided for macOS, Windows, and Linux. The latest binaries were built on macOS 12, Windows 10, and Red Hat Enterprise Linux (RHEL) 7 and 8. The Linux binaries usually will not work on other Linux distributions,...
Home
Page • The Dakota project delivers both state-of-the-art research and robust, usable software for optimization and UQ. Broadly, the Dakota software's advanced parametric analyses enable design exploration, model calibration, risk analysis, and quantification of margins and uncertainty with computational models. Read More.
Installation
Page • Unpack and Configure Installation instructions for pre-built binaries for macOS, Windows, and Red Hat Enterprise Linux 7 and 8 are below. For other platforms, you will need to build Dakota from source using the instructions in our User's Manual. Get the Dakota binary package for your platform.Extract Dakota zip or...
License
Page • Licensing terms affecting the use, copying, distribution, and modification of Dakota and its related Dakota GUI are summarized here. These terms potentially affect contributions submitted for inclusion in the Dakota project; please review the terms of contribution before authoring. Dakota GUI Dakota Graphical User Interface (Dakota GUI): Copyright 2017 Sandia...
Manuals
Page • Manuals for release 6.17 and later are available at Dakota's documentation site. Prior to release 6.17, Dakota documentation was divided into separate manuals: User's, Reference, Theory, and Developer's. These are available for download below for several previous releases. User's ManualDownload Reference ManualDownload Theory ManualDownload Developer's ManualDownload User's ManualDownload Reference ManualDownload...
Packages
Page • Sandia-Developed Numerical Libraries Dakota utilizes the following Sandia-developed optimization, design of experiments, uncertainty quantification, and surrogate modeling libraries: DDACE (design and analysis of computer experiments; available under GNU LGPL); DDACE additionally uses the MARS and OA packages from StatLib (conditions of use)HOPSPACK (Hybrid Optimization Parallel Search PACKage, which supplies a...
PECOS
Page • PECOS (Parallel Environment for Creation Of Stochastics) is a Dakota support library, initially created to generate samples of random fields (RFs) and stochastic processes (SPs) from a set of user-defined power spectral densities (PSDs). The RF/SP may be either Gaussian or non-Gaussian and either stationary or nonstationary, and the resulting sample is intended for run-time query...
Publications
Page • Eldred, M.S., Uncertainty Quantification Tutorial for Computer Scientists, ASCR Exascale Research PI Meeting, Annapolis, MD, Oct. 13, 2011. Giunta, A.A., Eldred, M.S., Field, R.V., and Swiler, L.P., Sensitivity Analysis and Uncertainty Quantification Short Course, AFRL Wright Labs, Dayton, OH, May 12-14, 2009. Giunta, A.A. and Eldred, M.S., Sensitivity Analysis and Uncertainty Quantification...
Retirement of dakota-users
Post, February 15, 2023 • The Dakota team is excited to announce that user support has moved to GitHub Discussions. The dakota-users listserv is now officially retired. Although dakota-users has served our community well over the years, GitHub Discussions has numerous features that we believe warrant this change. Some of these include: Searchable historyThreaded discussionsmarkdown formattingLaTeX style mathematical expressionsVoting, best...
Russell Hooper
Staff Page • R&D S&E, Computer Science. Biography Research Interests: Computational Science R&DPasma Physics Modeling & SimulationEfficient numerical algorithms for coupled multiphysics & code couplingHigh performance & scientific computing Code Development: Trilinos (http://trilinos.org)DAKOTA (https://dakota.sandia.gov)Aleph plasma physics SIERRAAlegraXyceVERA (The Virtual Environment for Reactor Applications) (https://www.casl.gov/vera) Education 2001 Ph.D. Chemical Engineering, University of Minnesota, Minneapolis,...
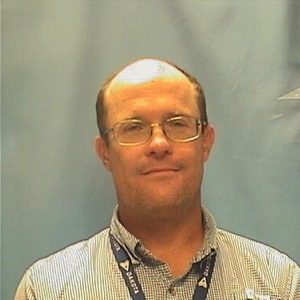
Screencasts
Page • Introduction to Dakota Sensitivity Analysis
Surfpack
Page • Overview NOTE: As of September 2008, Surfpack is no longer under active development. Surfpack will continue to be maintained as part of the Dakota software toolkit. Surfpack is a general-purpose software library of multidimensional function approximation methods for applications such as data visualization, data mining, sensitivity analysis, uncertainty quantification, and...
Team
Page • The success of the Dakota project depends on contributions from many people who share many roles, including algorithms research, software development, documentation, business management, DevOps, user support, and more. The current roster of active Dakota team members is shown below. Past contributors are listed on the Contributors page with our...
Results 1–25 of 28